Variational Relational Point Completion Network
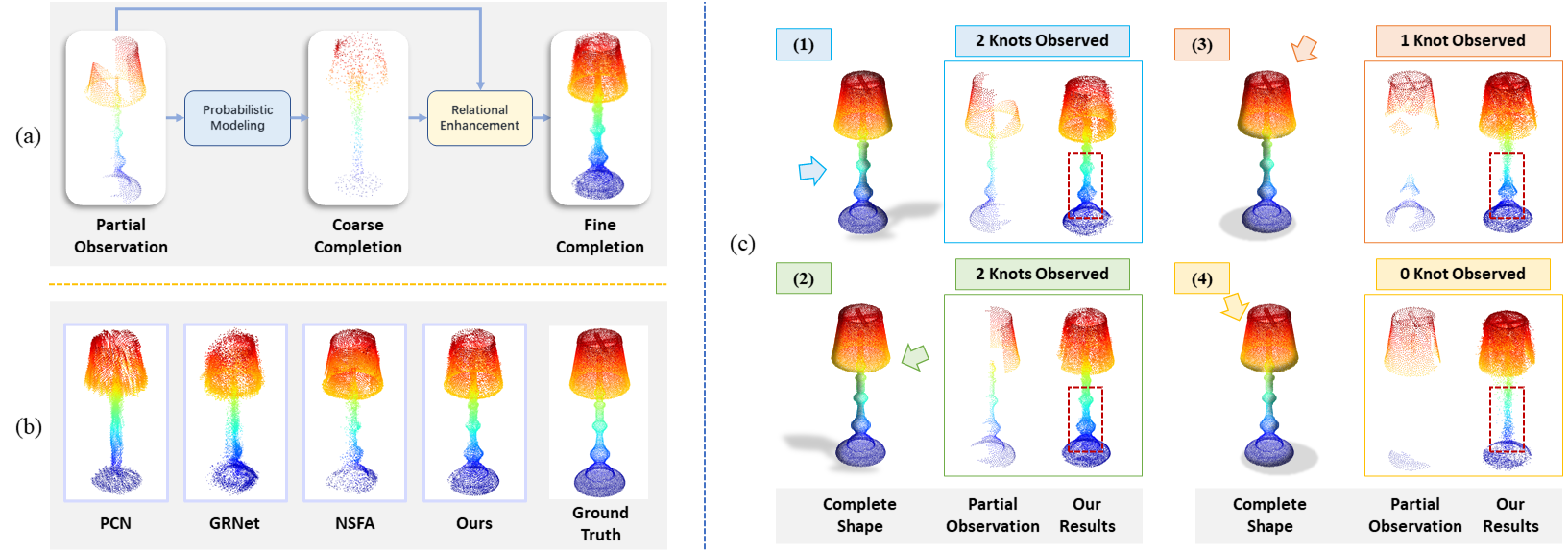
(a) VRCNet performs shape completion with two consecutive stages: probabilistic modeling and relational enhancement. (b) Qualitative Results show that VRCNet generates better shape details than the other works. (c) Our completion results conditioned on partial observations. The arrows indicate the viewing angles. In (1) and (2), 2 knots are partially observed for the pole of the lamp, and hence we generate 2 complete knots. In (3), only 1 knot is observed, and then we reconstruct 1 complete knot. If no knots are observed (see (4)), VRCNet generates a smooth pole without knots.
News
2021-07-12 The submission on Codalab starts!
2021-07-10 Two benchmarks, Single-View Point Cloud Completion and
Partial-to-Partial Point Cloud Registration
based on the MVP database have been released.
2021-07-09 An open-source toolbox for Point Cloud Completion and Registration, MVP codebase, has been released.
2021 The MVP challenges will be hosted in the ICCV2021 Workshop: Sensing, Understanding and Synthesizing Humans.
Abstract
Real-scanned point clouds are often incomplete due to viewpoint, occlusion, and noise. Existing point cloud completion methods tend to generate global shape skeletons and hence lack fine local details. Furthermore, they mostly learn a deterministic partial-to-complete mapping, but overlook structural relations in man-made objects. To tackle these challenges, this paper proposes a variational framework, Variational Relational point Completion network (VRCNet) with two appealing properties: 1) Probabilistic Modeling. In particular, we propose a dual-path architecture to enable principled probabilistic modeling across partial and complete clouds. One path consumes complete point clouds for reconstruction by learning a point VAE. The other path generates complete shapes for partial point clouds, whose embedded distribution is guided by distribution obtained from the reconstruction path during training. 2) Relational Enhancement. Specifically, we carefully design point self-attention kernel and point selective kernel module to exploit relational point features, which refines local shape details conditioned on the coarse completion. In addition, we contribute a multi-view partial point cloud dataset (MVP dataset) containing over 100,000 high-quality scans, which renders partial 3D shapes from 26 uniformly distributed camera poses for each 3D CAD model. Extensive experiments demonstrate that VRCNet outperforms state-of-the-art methods on all standard point cloud completion benchmarks. Notably, VRCNet shows great generalizability and robustness on real-world point cloud scans.
Method Highlight
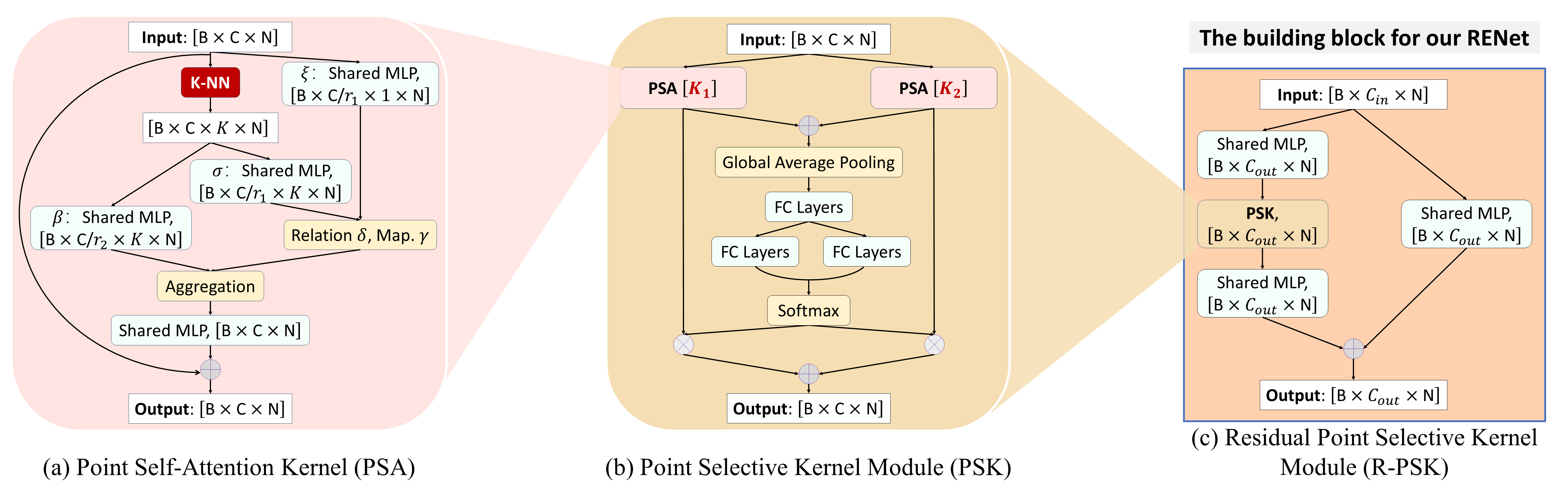
Our proposed point kernels. (a) Our PSA adaptively aggregate neighboring point features. (b) Using selective kernel unit, our PSK can adaptively adjust receptive fields to exploit and fuse multi-scale point features. (c) By adding a residual connection, we construct our RPSK that is an important building block for our RENet.
MVP Dataset
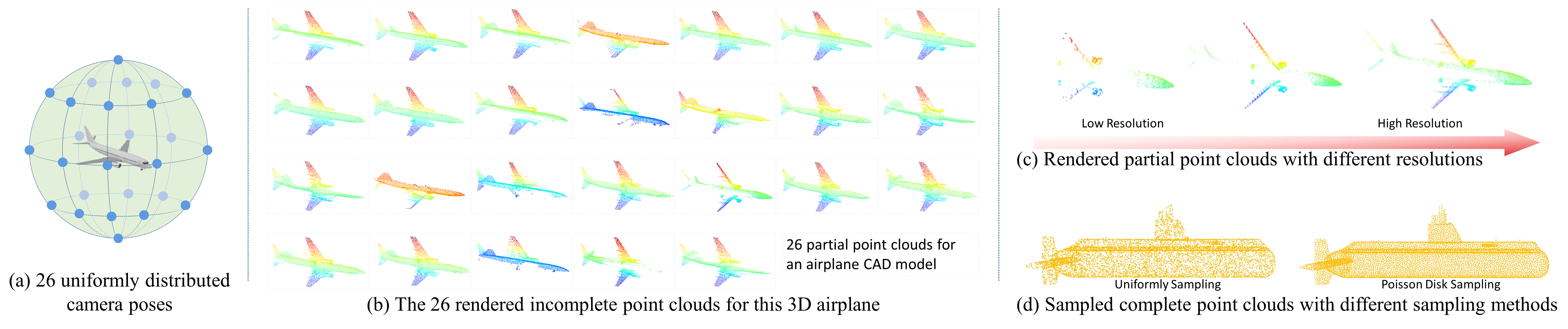
Multi-View Partial (MVP) Point Cloud Dataset. (a) shows an example for our 26 uniformly distributed camera poses on a unit sphere. (b) presents the 26 partial point clouds for the airplane from our uniformly distributed virtual cameras. (c) compares the rendered incomplete point clouds with different camera resolutions. (d) shows that Poisson disk sampling generates complete point clouds with a higher quality than uniform sampling. Download: [Google Drive], [Dropbox].
Citation
@article{pan2021variational, title={Variational Relational Point Completion Network}, author={Pan, Liang and Chen, Xinyi and Cai, Zhongang and Zhang, Junzhe and Zhao, Haiyu and Yi, Shuai and Liu, Ziwei}, journal={arXiv preprint arXiv:2104.10154}, year={2021} }